Private AI Collaborative Research Institute
Intel, in collaboration with Avast and Borsetta, launched the Private AI Collaborative Research Institute to advance and develop technologies in privacy and trust for decentralized AI.
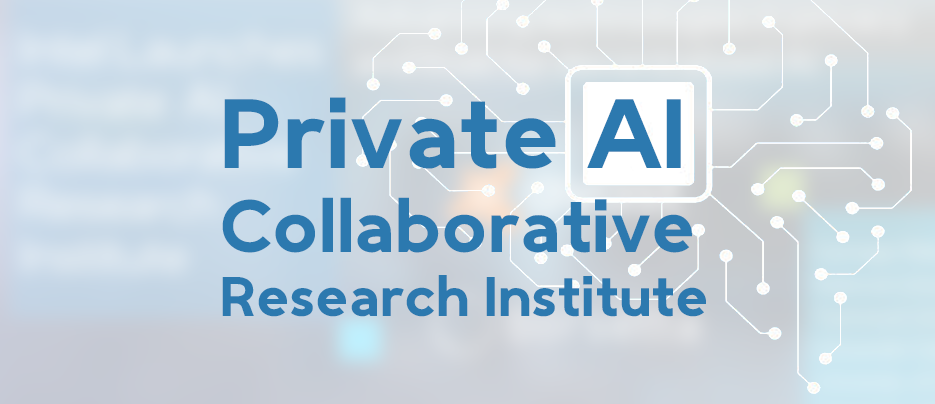
The Private AI Collaborative Research Institute selected the first nine institute-supported research projects, distributed among eight universities worldwide. The Univeristy of Würzburg is one of them.
The industry is trending toward intelligent edge systems. Algorithms such as neural networks and distributed ledgers gain traction at the edge on the device level without reliance on cloud infrastructure. To be effective, this requires vast amounts of data that is often sensed at the edge, such as vehicle routing, industrial monitoring, security threat monitoring, or search term predictions.
The Private AI Collaborative Research Institute will focus its efforts on overcoming five main challenges:
- Training data is decentralized in isolated silos and often inaccessible.
- Today’s solutions are insecure and require a single trusted data center.
- Centralized models become obsolete quickly.
- Centralized compute resources are costly and throttled by communication and latency.
- Federated machine learning (FL) is limited.
While FL can access data at the edge, it cannot reliably guarantee privacy and security. Here is where Prof. Dr. Dmitrienko, head of the research group Secure Software Systems at the University of Würzburg, will contribute towards designing a framework for Federated Learning (FL) resilient against security and privacy threats. The design will incorporate security mechanisms against various attack vectors such as data poisoning and model inference. It will focus on integration of hardware-assisted security and trusted execution environments of varying capabilities for achieving improved privacy and integrity guarantees.
More information at private-ai.org
People involved: Prof. A. Dmitrienko, Christoph Sendner, and Torsten Krauß.
Collaboration with Prof. Farinaz Koushanfar, UC San-Diego is also supported by BaCaTeC.
Publications
-
CrowdGuard: Federated Backdoor Detection in Federated Learning. in the Network and Distributed System Security Symposium (NDSS) (2024).
- [ BibTeX ]
- [ Download ]
- [ BibSonomy-Post ]
-
Security of NVMe Offloaded Data in Large-Scale Machine Learning. in European Symposium on Research in Computer Security (ESORICS) (2023).
- [ BibTeX ]
- [ URL ]
- [ Download ]
- [ BibSonomy-Post ]
-
Avoid Adversarial Adaption in Federated Learning by Multi-Metric Investigations. in ArXiv | arXiv.2306.03600 (2023).
- [ BibTeX ]
- [ URL ]
- [ Download ]
- [ BibSonomy-Post ]
-
Close the Gate: Detecting Backdoored Models in Federated Learning based on Client-Side Deep Layer Output Analysis. in ArXiv | arXiv:2210.07714 (2022).
- [ BibTeX ]
- [ URL ]
- [ BibSonomy-Post ]
-
Vision: Challenges & Opportunities. in Vision Paper of the Private AI Collaborative Research Institute (2021).
- [ BibTeX ]
- [ Download ]
- [ BibSonomy-Post ]
-
Detection of Software Vulnerabilities in Smart Contracts using Deep Learning. Thesis; University of Würzburg. (2020, October).
- [ Abstract ]
- [ BibTeX ]
- [ Download ]
- [ BibSonomy-Post ]